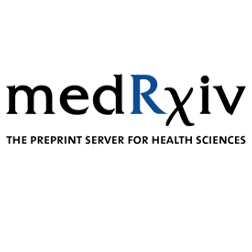
Quantifying the impact of social activities on SARS-CoV-2 transmission using Google mobility reports
We developed a state-space model to investigate which social behaviours had biggest impact on the spread of SARS-CoV-2. The analyses were based on reported hospitalizations, together with information on vaccinations, weather data, virus strains and, most importantly, Google mobility reports on 4 different types of social activities. While our new approach is general, we studied Sweden and Norway on a regional level over 75 weeks, and the major regions of Berlin and Bavaria in Germany over 10 months. Most results are shared for all three countries: Activity in four social settings explain between 40-60% of all infections; Public transport appears as an important setting for infections in all countries; and the transmission potential drops by 40-50% during the summer as compared to the winter peak. However, the analyses for Germany differ in that Retail and recreation is the other setting dominating transmission whereas it is contacts at the Workplace in Norway and Sweden, showing how our model is able to adapt to specific cases. Transmissions not captured by the Google data may happen in other settings, in particular in households. The statistical model has a deterministic time and region specific transmission rate with an additive component for the four Google settings, and a multiplicative part taking seasonality and circulating virus strains into account. Inference is performed in a Bayesian setting using Stan. ### Competing Interest Statement The authors have declared no competing interest. ### Funding Statement This work was supported by the Nordforsk project 105572. AF and HKB were also supported by BigInsight, the Norwegian Research Council project number 237718. AF was also supported by Integreat, the Norwegian Research Council project number 332645. ### Author Declarations I confirm all relevant ethical guidelines have been followed, and any necessary IRB and/or ethics committee approvals have been obtained. Yes The details of the IRB/oversight body that provided approval or exemption for the research described are given below: The study used publicly available data on the number the number of Covid-19 hospitalizations, vaccination numbers, and share of virus variants as provided by the Public health agencies of the considered countries (Sweden, Norway, and Germany). The finest level of aggregation was weekly (cumulative) numbers at county/federal state level. Data was obtained from the following web ressources: https://www.fhi.no/en/id/corona/coronavirus/daily-reports/daily-reports-COVID19/Data https://www.socialstyrelsen.se/statistik-och-data/statistik/statistik-om-covid-19/ https://github.com/robert-koch-institut/COVID-19-Hospitalisierungen\_in\_Deutschland/ I confirm that all necessary patient/participant consent has been obtained and the appropriate institutional forms have been archived, and that any patient/participant/sample identifiers included were not known to anyone (e.g., hospital staff, patients or participants themselves) outside the research group so cannot be used to identify individuals. Yes I understand that all clinical trials and any other prospective interventional studies must be registered with an ICMJE-approved registry, such as ClinicalTrials.gov. I confirm that any such study reported in the manuscript has been registered and the trial registration ID is provided (note: if posting a prospective study registered retrospectively, please provide a statement in the trial ID field explaining why the study was not registered in advance). Yes I have followed all appropriate research reporting guidelines, such as any relevant EQUATOR Network research reporting checklist(s) and other pertinent material, if applicable. Yes All data produced in the present study are available upon reasonable request to the authors. Code and data for a central part of the analysis are available at: https://github.com/FelixGuenther/nordic\_behavior\_public/ Publicly available data on COVID-19 hospitalization counts were obtained from NOR: https://www.fhi.no/en/id/corona/coronavirus/daily-reports/daily-reports-COVID19/Data SWE: https://www.socialstyrelsen.se/statistik-och-data/statistik/statistik-om-covid-19/ GER: https://github.com/robert-koch-institut/COVID-19-Hospitalisierungen\_in\_Deutschland/ [https://github.com/FelixGuenther/nordic\_behavior\_public/][1] [1]: https://github.com/FelixGuenther/nordic_behavior_public/
- Günther, F., Brustad, H. K., Frigessi, A., & Britton, T. (2024). Quantifying the impact of social activities on SARS-CoV-2 transmission using Google mobility reports. https://doi.org/10.1101/2024.01.03.24300755
この論文は、SARS-CoV-2(新型コロナウイルス)の感染拡大にどの社会活動が最も大きな影響を与えているのかを調べるために状態空間モデルを開発したものである。解析は、報告された入院件数に加え、ワクチン接種情報、気象データ、ウイルス株、そして最も重要な点として、グーグルが提供する4種類の社会活動別の移動履歴データに基づいている。
この新しい手法は汎用性があるものの、本研究ではスウェーデンとノルウェーを75週にわたって地域レベルで、ドイツのベルリンとバイエルンの主要地域を10カ月以上にわたって調査した。
3カ国共通の主な結果は以下の通りである。
- 4種類の社会活動における活動量は、全体の感染件数の40~60%を説明している。
- 公共交通機関は、どの国においても感染拡大にとって重要な設定因子であることが示された。
- ウイルス感染の拡大ピークである冬と比べると、夏場の感染拡大 potential(潜在力)は40~50%低下する。
ただし、ドイツの解析結果は他国と異なっており、感染拡大に最も影響しているのは「小売店や娯楽施設」での活動であるのに対し、ノルウェーとスウェーデンでは「職場」での接触が主な要因となっている。この点からも、我々のモデルは特定の事例に適応できる柔軟性を備えていることが示される。
なお、グーグルのデータで捉えきれない感染経路としては、家庭内での接触などが考えられる。
このモデルは、決定論的な時間と地域ごとの感染率を持ち、4種類のグーグルのデータを足し合わせた成分と、季節変動や流行しているウイルス株を考慮した乗算成分から構成されている。推論は、Stanと呼ばれるソフトウェアを用いたベイズ統計手法で行われている。